How to read survey results: what most analysts miss in 2025
Discover why traditional survey analysis fails and how AI-driven approaches transform data collection into meaningful business insights.
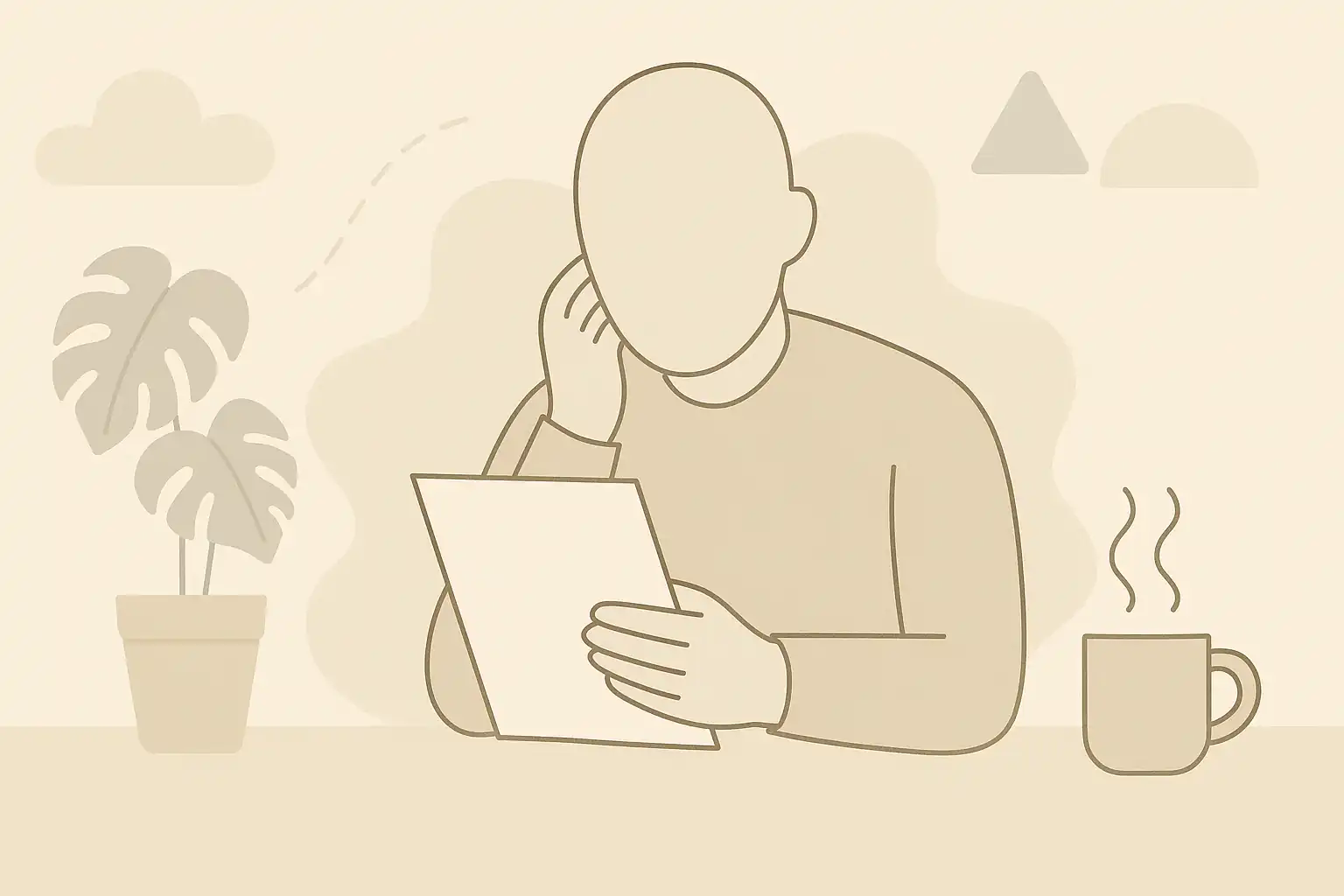
Survey data sits unused in spreadsheets because organizations collect numbers without context. Understanding how to extract meaningful insights requires a fundamental shift in approach.
Marketing expert Lihong Hicken demonstrates that effective survey analysis now depends on capturing both quantitative metrics and qualitative explanations simultaneously.
Short on time?
Here's a table of contents for quick access:
- The three types of survey data that matter
- Why traditional survey methods create analysis bottlenecks
- How conversational AI transforms data collection
- Step-by-step implementation of AI-driven surveys
- Test results: comparing three analysis methods
The three types of survey data that matter
Survey responses now fall into distinct categories with varying degrees of usefulness:
- Quantitative data - numerical responses through ratings and selections
- Qualitative data - contextual explanations behind the numbers
- AI conversations - adaptive exchanges that reveal deeper motivations
Organizations focusing solely on quantitative metrics like customer satisfaction scores miss critical context. A "3-star" rating without explanation becomes a data point without direction.
The central issue isn't analysis techniques but the fundamental limitations of the data being collected.
Why traditional survey methods create analysis bottlenecks
Traditional survey platforms create three specific roadblocks to actionable insights:
First, they separate quantitative and qualitative questions, making correlation between scores and explanations difficult to establish.
Second, they offer no mechanism for follow-up questions when responses need clarification, leaving potential insights unexplored.
Third, they produce disconnected data sets that require manual interpretation, increasing analysis time and introducing potential bias.
Testing reveals that uploading quantitative-only data to analysis tools produces correlations without causation. The output might show "AI tool adoption links to higher satisfaction" but fails to explain why this connection exists.
How conversational AI transforms data collection
AI-powered survey systems replace static forms with dynamic exchanges that adapt based on responses.
In practice, this means when a respondent gives a neutral "3" rating about a flight experience, the AI immediately asks "What made your experience neutral?" When the passenger mentions "slow boarding," the system follows up with specific questions about wait times and luggage handling.
This approach offers measurable advantages:
- It captures ratings and explanations in a single connected interaction
- It provides structured outlets for respondent frustrations
- It automatically categorizes responses into actionable themes
Step-by-step implementation of AI-driven surveys
Setting up AI-powered surveys requires a different workflow:
- Define specific learning objectives ("Understand key factors influencing workplace happiness")
- Allow AI to generate question sets based on stated objectives
- Incorporate multiple response formats (choice, ranking, open-ended)
- Distribute through multiple channels (links, QR codes, embeds)
- Use automated theme detection rather than manual coding
Platforms like theyset.io now offer free options for organizations to test this approach without significant investment.
Test results: comparing three analysis methods
Controlled testing of different analysis methods reveals significant performance gaps:
Quantitative-only analysis produced visual patterns but lacked explanatory context, generating graphs that showed "what" but not "why."
Qualitative-only analysis identified themes (team support, work flexibility) but couldn't quantify their prevalence or relative importance.
AI conversational analysis automatically grouped responses into categorized themes with frequency counts and specific examples, creating both measurement and context.
The most revealing finding: organizations cannot take meaningful action on survey data without understanding both the metrics and the motivations behind them.
Converting feedback into focused improvements
Survey effectiveness ultimately depends on response quality rather than quantity. When respondents engage in conversational exchanges, they provide more specific and actionable information.
Organizations now face a clear choice: continue collecting isolated data points or implement systems that capture both problems and potential solutions.
The most successful survey programs in 2025 will focus on gathering integrated data sets that connect what's happening with why it's happening, creating clear roadmaps for organizational improvement.
Most organizations won't adapt their survey methods. But those who embrace conversational approaches will transform feedback from metrics into meaningful change.
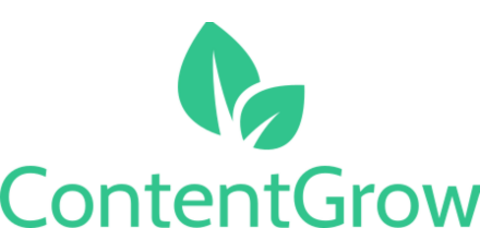